AI and Data Protection: 7 Key Challenges and Solutions in the Digital Age
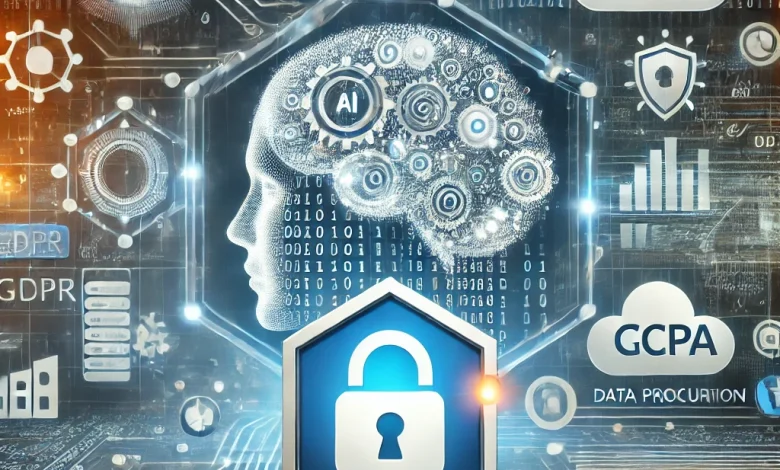
Table of Contents
I. Introduction
In the digital age, artificial intelligence (AI) has become a cornerstone of innovation, driving advancements in healthcare, finance, and entertainment. AI’s ability to process vast amounts of data has enabled transformative applications, but it has also raised significant concerns about data privacy. As AI systems increasingly rely on data to function, safeguarding this information has become more critical than ever.
The importance of data in AI systems cannot be overstated. From training machine learning models to generating insights, data forms the backbone of AI. However, the growing concern for data protection stems from the risks associated with data misuse, bias, and security breaches. This article explores the complex interplay between AI and data protection, shedding light on the challenges and strategies to ensure ethical and secure AI development.
II. The Intersection of AI and Data Protection
AI systems are inherently data-driven, leveraging algorithms to analyze, predict, and make decisions. This dependency on data, however, introduces unique challenges to privacy.
How AI Relies on Data
AI functions by ingesting and processing data to identify patterns, make predictions, and learn continuously. This data ranges from user preferences and behavioral patterns to sensitive personal information. For example, AI-driven healthcare systems use patient records to enhance diagnostic accuracy. However, the reliance on such data necessitates robust data protection measures to prevent misuse.
Challenges Posed by AI
AI’s reliance on data brings about privacy risks, including unauthorized data access, potential misuse, and lack of transparency in how data is processed. The complexity of AI algorithms often makes it difficult for individuals to understand how their data is being utilized.
Regulatory frameworks are essential to address these concerns. Governments worldwide are enacting laws to ensure AI systems adhere to privacy standards. At the same time, ethical considerations play a pivotal role in determining how data is collected, processed, and stored responsibly.
III. Key Data Protection Challenges Posed by AI
AI’s extensive data requirements introduce several challenges that must be addressed to protect user privacy effectively.
Data Collection and Consent Issues
AI systems often rely on mass data gathering, raising concerns about informed consent.
- Mass Data Gathering: AI systems analyze data from various sources, often without explicit user consent. This practice can lead to privacy violations.
- Informed Consent: Obtaining meaningful consent becomes complex as users may not fully understand the implications of sharing their data.
- Transparency Concerns: Users frequently remain unaware of how their data is processed, which erodes trust in AI systems.
Data Bias and Discrimination
AI’s reliance on data introduces the risk of bias, which can lead to discriminatory outcomes.
- Impact of Biased Data: When AI systems are trained on biased datasets, they can perpetuate inequality. For example, biased hiring algorithms may disadvantage certain demographics.
- Real-World Examples: AI-driven credit scoring systems have been found to disproportionately reject loan applications from minority groups.
- Mitigating Bias: Responsible data handling practices, such as diverse dataset inclusion and regular bias audits, can help minimize bias.
Security Vulnerabilities in AI Systems
AI systems are susceptible to cyber threats, making data protection paramount.
- Cyber Threats: Hackers may exploit vulnerabilities in AI systems to access sensitive data.
- AI in Cybersecurity: While AI can detect and prevent security breaches, its own vulnerabilities need to be addressed.
- Encryption and Anonymization: Robust encryption and anonymization techniques can enhance data security.
Automated Decision-Making and Accountability
Automated AI decisions can lack human oversight, raising ethical and legal concerns.
- Lack of Oversight: AI decisions without human intervention can lead to unfair outcomes.
- Legal Implications: AI-driven decisions, such as loan approvals or medical diagnoses, can have significant consequences, necessitating accountability.
- Explainability: Ensuring transparency in AI decision-making builds trust and ensures fairness.
Cross-Border Data Transfers and Compliance
The global nature of AI poses challenges in complying with varying data protection regulations.
- Global Deployment Challenges: AI systems deployed across borders must navigate different legal requirements.
- International Regulations: Compliance with laws such as GDPR and CCPA is essential for AI systems.
- Data Sovereignty: Jurisdictional concerns arise when data is transferred across countries.
IV. Legal and Regulatory Landscape
Understanding the legal framework is crucial for ensuring AI development aligns with data protection requirements. Governments and regulatory bodies worldwide are implementing stringent laws to safeguard personal data and address the challenges posed by AI-driven systems.
Major Data Protection Laws
- General Data Protection Regulation (GDPR):
- Introduced by the European Union (EU), GDPR is one of the most comprehensive data protection laws globally.
- It mandates strict requirements for data processing, including obtaining explicit user consent, ensuring data minimization, and providing transparency in data handling.
- Companies must implement measures such as the right to erasure (right to be forgotten), data portability, and appointing Data Protection Officers (DPOs) for oversight.
- Non-compliance can result in hefty fines of up to €20 million or 4% of annual global turnover.
- California Consumer Privacy Act (CCPA):
- Enacted in California, the CCPA grants consumers significant control over their personal information collected by businesses.
- It requires businesses to disclose the types of data collected, provide opt-out options for data selling, and ensure consumers can request deletion of their data.
- The CCPA also introduced provisions for consumers to sue businesses in the event of a data breach, increasing accountability.
- The subsequent California Privacy Rights Act (CPRA) expanded CCPA’s provisions by introducing stricter enforcement and new consumer rights.
- India’s Personal Data Protection Bill (PDPB):
- India’s PDPB aims to regulate the processing of personal data by government and private entities while ensuring user consent and data localization requirements.
- It emphasizes the need for explicit consent, data breach reporting, and imposing restrictions on cross-border data transfers.
- Non-compliance may result in severe penalties and legal action.
- China’s Personal Information Protection Law (PIPL):
- China’s PIPL is a comprehensive data protection law that imposes strict regulations on data processing, cross-border transfers, and data security.
- It requires companies to seek explicit user consent, ensure data security measures are in place, and comply with stringent reporting requirements.
- Violators face heavy fines and potential suspension of operations within China.
- Other Global Regulations:
- Various other countries, including Brazil (LGPD), Australia, and Canada, have enacted data protection laws addressing AI-related privacy challenges.
- Each regulation emphasizes transparency, user rights, and accountability in data handling.
Key Compliance Requirements for AI Systems
To ensure compliance with these legal frameworks, AI systems must adhere to several key requirements:
- Data Minimization:
- AI developers should collect and process only the data necessary for the intended purpose, avoiding excessive data retention and minimizing privacy risks.
- User Rights:
- Individuals must have the right to access their data, request its deletion, correct inaccuracies, and transfer data between service providers (data portability).
- Impact Assessments:
- AI projects must undergo Data Protection Impact Assessments (DPIAs) to evaluate risks associated with data processing and ensure compliance with regulatory standards.
- Accountability and Governance:
- Organizations must establish governance frameworks, appoint data protection officers, and regularly audit AI systems to detect compliance gaps.
- Security Measures:
- Implementation of encryption, anonymization, and strict access controls to safeguard sensitive data against unauthorized access and breaches.
Recent Legal Cases and Precedents
Several high-profile legal cases have highlighted the importance of compliance with data protection laws:
This case underscores the importance of clear and explicit consent for data processing in AI systems.
Google’s GDPR Fine (France):
Google was fined €50 million by the French data protection authority (CNIL) for lack of transparency and inadequate user consent mechanisms in targeted advertising practices.
V. Strategies to Enhance AI Data Protection
Enhancing AI and data protection requires a comprehensive, multifaceted approach that integrates privacy-focused strategies, cutting-edge technologies, and collaboration among various stakeholders. As AI continues to evolve, organizations must prioritize robust data protection measures to safeguard user information and maintain compliance with global regulations. ai and data protection
Privacy by Design in AI Systems
One of the most effective strategies to ensure AI and data protection is adopting the Privacy by Design approach. This involves embedding privacy measures into AI systems from the initial development stages rather than treating them as an afterthought.
- Ethical Design:
AI developers must consider ethical principles to ensure that AI systems handle data responsibly. Ethical AI design incorporates privacy-enhancing features such as data anonymization and access control mechanisms. Prioritizing ethics ensures that AI respects user privacy and regulatory standards. - Proactive Monitoring and Auditing:
Regular monitoring and auditing of AI systems are crucial to maintaining compliance with AI and data protection laws. Auditing helps identify potential vulnerabilities and ensures that data processing aligns with privacy policies and legal frameworks.
Implementing Privacy by Design not only enhances AI and data protection but also builds trust among consumers and regulatory bodies by demonstrating a proactive commitment to privacy.
Advanced Data Protection Technologies
Technological advancements play a pivotal role in strengthening AI and data protection, providing innovative solutions to protect sensitive information without compromising AI capabilities.
- Differential Privacy:
This technique ensures that AI models can analyze large datasets without revealing individual data points. It adds noise to data queries, providing statistical insights while maintaining user anonymity, thus enhancing AI and data protection. - Federated Learning:
Federated learning allows AI models to train across decentralized data sources without transferring raw data to central servers. This technique minimizes exposure to potential security threats, reducing privacy risks and improving AI and data protection. - Homomorphic Encryption:
Homomorphic encryption enables AI systems to perform computations on encrypted data without decrypting it first. This ensures sensitive data remains protected throughout processing, making it an essential tool for ensuring AI and data protection.
By leveraging these technologies, businesses can significantly enhance their AI systems’ security and compliance with evolving data protection laws. ai and data protection
Transparency and Explainability in AI Systems
Transparency and explainability are critical factors in promoting user trust and ensuring AI and data protection. Users and regulators must understand how AI systems process their data and the rationale behind automated decisions.
- Developing Interpretable AI Models:
AI models should be designed in a way that allows users to comprehend their decision-making processes. Interpretability ensures that users can hold AI systems accountable, fostering trust in AI and data protection measures. - Enhancing Explainability:
AI systems should provide clear and concise explanations regarding data usage, processing techniques, and privacy safeguards. Transparency reports and privacy policies should communicate how personal data is handled, strengthening compliance with AI and data protection regulations.
Greater transparency helps build user confidence and ensures AI systems operate within ethical and legal boundaries.
Collaboration Between Stakeholders
Collaboration among stakeholders is essential for creating a secure and ethical AI ecosystem that upholds AI and data protection standards. This includes partnerships between policymakers, technology companies, and consumers. ai and data protection
- Role of Policymakers and Tech Companies:
Governments and technology firms must work together to establish comprehensive regulatory frameworks that guide AI development while ensuring data privacy. Policymakers can enforce compliance, while tech companies can innovate within legal constraints to enhance AI and data protection. - Consumer Awareness and Education:
Educating users about their data privacy rights is crucial for ensuring effective AI and data protection. Awareness campaigns can inform users about their rights under regulations like GDPR and CCPA, empowering them to make informed decisions regarding their data. - Industry Standards and Best Practices:
Establishing industry-wide best practices and certifications can help organizations align their AI systems with global data protection standards. Adopting frameworks such as ISO 27001 for information security management contributes to improving AI and data protection.
Encouraging collaboration ensures that all stakeholders contribute to creating a secure AI landscape that prioritizes privacy and ethical data usage.
Implementing Robust Security Measures
A critical aspect of AI and data protection is implementing strong security protocols to prevent unauthorized access and data breaches. Organizations should focus on:
- Data Encryption: Ensuring all sensitive AI data is encrypted during storage and transmission to prevent unauthorized access.
- Access Controls: Implementing strict user access permissions and multi-factor authentication (MFA) to safeguard AI data.
- Incident Response Plans: Developing and regularly testing incident response strategies to address potential data breaches effectively.
These security measures play a fundamental role in ensuring compliance with data protection laws and maintaining user trust.
Continuous Improvement and Compliance Monitoring
AI systems and data protection strategies must evolve to address emerging threats and regulatory changes. Regular compliance assessments and updates help organizations stay ahead in the rapidly changing landscape of AI and data protection. ai and data protection
- Conducting Privacy Impact Assessments (PIAs): Organizations should perform periodic PIAs to evaluate how AI systems impact user privacy and address potential risks.
- Adopting Adaptive AI Systems: AI systems should be designed to adapt to new privacy regulations and evolving data protection requirements.
By continuously refining data protection strategies, businesses can ensure their AI systems remain compliant and secure over time.
VI. Future Trends and Challenges
The intersection of AI and data protection is evolving, presenting new challenges and opportunities.
- Regulatory Evolution: Anticipate updates to existing laws and efforts for global AI governance.
- Ethical AI: Focus on fairness and transparency in AI systems.
- Emerging Technologies: Innovations like blockchain and quantum computing may redefine data privacy.
Conclusion
The integration of artificial intelligence (AI) with vast amounts of data offers immense opportunities across various industries, but it also raises significant concerns regarding data protection. As AI systems become more advanced, the need for robust data protection strategies becomes increasingly critical to ensure compliance with global regulations, ethical considerations, and user trust.
To address the challenges posed by AI’s reliance on data, organizations must adopt a multifaceted approach that includes privacy-by-design principles, advanced data protection technologies, transparency, and collaboration among stakeholders. Ensuring compliance with key regulations such as GDPR, CCPA, PDPB, and PIPL is crucial to maintaining legal and ethical AI operations.
Looking ahead, the landscape of AI and data protection will continue to evolve, driven by advancements in technology, stricter regulatory requirements, and growing public awareness of data privacy rights. Organizations that prioritize data protection today will be better positioned to leverage AI’s full potential while maintaining the trust and confidence of their users.
By embracing ethical AI practices and continuously refining data protection strategies, businesses can navigate the complex intersection of AI and data privacy, ensuring sustainable growth in the digital age.
Frequently Asked Questions (FAQs)
1. Why is data protection important in AI systems?
Data protection is crucial in AI systems to prevent unauthorized access, data misuse, and privacy violations. AI systems rely on large datasets to function effectively, and without proper safeguards, sensitive user information can be exposed to security threats and regulatory non-compliance.
2. What are the biggest data protection challenges posed by AI?
The key challenges include mass data collection without proper user consent, data bias leading to discrimination, security vulnerabilities, lack of transparency in automated decision-making, and difficulties in complying with international data protection laws.
3. How does GDPR impact AI and data protection?
The GDPR imposes strict data protection requirements, including data minimization, explicit user consent, transparency, and accountability. AI systems operating within the EU or handling EU citizens’ data must comply with GDPR regulations to avoid hefty fines and legal actions.
4. What technologies can enhance AI data protection?
Advanced technologies such as differential privacy, federated learning, and homomorphic encryption help enhance data protection in AI by ensuring that sensitive information remains secure while still allowing AI systems to analyze and process data effectively.
5. How can businesses ensure compliance with global data protection laws?
Businesses can ensure compliance by implementing data governance frameworks, conducting regular privacy impact assessments (PIAs), appointing Data Protection Officers (DPOs), using encryption techniques, and staying informed about evolving regulatory changes across different regions.
6. What is Privacy by Design in AI development?
Privacy by Design is a proactive approach that integrates data protection measures into AI systems from the very beginning of their development lifecycle. It ensures that privacy considerations are embedded into system architecture, reducing risks and enhancing compliance.
7. How can AI bias be mitigated to ensure fairness?
AI bias can be mitigated by using diverse and representative datasets, conducting regular audits to identify potential biases, implementing fairness-focused algorithms, and ensuring human oversight in AI decision-making processes.
8. What role do policymakers play in AI and data protection?
Policymakers are responsible for establishing legal frameworks and guidelines that ensure AI systems operate ethically and securely. They collaborate with technology companies to enforce compliance and protect consumer rights.
9. What are the risks of cross-border data transfers in AI systems?
Cross-border data transfers can pose legal and security challenges due to varying data protection laws across jurisdictions. Businesses must ensure compliance with regional regulations such as GDPR and PIPL and consider data localization measures when necessary.
10. What are the future trends in AI and data protection?
Future trends include stricter regulatory frameworks, increased focus on ethical AI, advancements in privacy-enhancing technologies, and the adoption of decentralized data processing techniques to minimize data exposure.